Enhancing Maize Disease Detection: A Comprehensive Study on Few-Shot Learning, Object Detection, and Synthetic Data Generation Using YOLOv5s-C3CBAM Model
Keywords:
Staple Crops, Disease Detection, Single Leaf Disorders, Unmanned Aerial Vehicles, Growth StagesAbstract
As the global population grows, ensuring food production, particularly of staple crops like maize, becomes crucial. Maize faces challenges from diseases impacting both yield and quality, threatening global food security. Traditional disease detection methods are inefficient, prompting a shift towards computer vision and machine learning. However, data scarcity hinders model training. This study explores few-shot learning to address this issue. In the context of maize in China, leaf diseases pose a significant threat, causing substantial economic losses. Current research focuses on single-leaf disorders, emphasizing the need for advanced detection algorithms, especially for complex diseases. Additionally, accurate plant counting during growth stages is vital for effective management, with computer vision-based approaches offering promising solutions, especially with Unmanned Aerial Vehicles (UAVs). Object detection technology, employing both one-stage and two-stage methods, plays a pivotal role in agricultural research. YOLOv5s emerges as an efficient model, demonstrating success in various applications, including disease detection and plant counting. The study introduces a comprehensive methodology involving dataset expansion, Cycle GAN for synthetic data, and the YOLOv5s-C3CBAM model for maize disease detection. Results indicate the superiority of the YOLOv5s-C3CBAM model, with improvements in mean average precision (mAP), recall, F1 score, and precision. The study delves into model comparisons, experimental parameters, and disease identification accuracy. The YOLOv5s-C3CBAM model achieves 83% mAP_0.5, outperforming other models. Limitations, such as focusing on three diseases, are acknowledged, and future directions involve building a more comprehensive dataset. The study introduces innovations in image generation using Cycle GAN and attention processes, addressing challenges in disease detection accuracy. Despite limitations, the YOLOv5s-C3CBAM model contributes to accurate crop disease diagnosis, serving as a reference for future agricultural research.
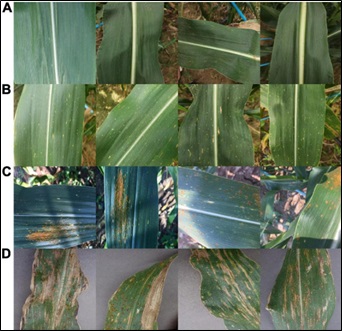